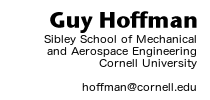
Reinforcement Learning with Human Teachers: Understanding How People Want to Teach Robots
Proceedings of the 15th IEEE International Symposium on Robot and Human Interactive Communication
Abstract
While Reinforcement Learning (RL) is not traditionally designed for interactive supervisory input from a human teacher, several works in both robot and software agents have adapted it for human input by letting a human trainer control the reward signal. In this work, we experimentally examine the assumption underlying these works, namely that the human-given reward is compatible with the traditional RL reward signal. We describe an experimental platform with a simulated RL robot and present an analysis of real-time human teaching behavior found in a study in which untrained subjects taught the robot to perform a new task.
We report three main observations on how people administer feedback when teaching a robot a task through Reinforcement Learning: (a) they use the reward channel not only for feedback, but also for future-directed guidance; (b) they have a positive bias to their feedback — possibly using the signal as a motivational channel; and (c) they change their behavior as they develop a mental model of the robotic learner. In conclusion, we discuss future extensions to RL to accommodate these lessons.